



How We Build With Design Partners
Monday, July 10, 2023
At Streamline, our mission is to unlock critical funding for climate tech to innovate, deploy and scale. Today, we help accelerate grant applications. To build the most delightful, trustworthy, and useful product, we’ve partnered with climate heroes — Noya, Perennial, and Vesta — actively facing the problem.
We’re excited to dive into our process with some key early learnings — one of which, is in order to build a trustworthy generative AI platform, it’s critical to remove magic ✨ — more on this below.
First - what is a design partner?
A design partner is an organization that helps guide the development of a product. You iterate closely, turning ideas into prototypes, and finally into a full product.
For our first batch of design partners, we looked for a few things:
Willingness to adopt new technology - A great design partner is excited about being on the ground floor, understands demos will break, and feel invested to improve the product.
Relevant experience - This will be feature dependent. In our case, this means having applied to and won government grants in the past.
Shared urgency - For this feature, having an upcoming grant opportunity was important as asking someone to jump on weekly calls for feedback is a big ask. Aligning ourselves around an upcoming grant creates a shared sense of urgency and commitment that allows us to get feedback and iterate quickly.
Ultimately, design partners are truly partners with a large stake in co-designing a solution. We know their time is valuable, so we structure our process in a way to make sure we make the best use of their time.
How do we run design partnerships?
We created a 3-phase process with our design partners - discover, prototype, build. Each stage lasted roughly 2 weeks, with weekly 45 min calls. Here’s roughly how we thought of the 3 stages:
Discover - We learn about how the customer solve these problems today and discover adjacent problems, if any. Here we identify the correct point of contact to run an effective process. (The Mom Test is an excellent read on this topic).
Prototype - Here we start showing high fidelity designs. Our founding designer (Deo) and CTO (Doug) work closely to develop prototypes and gauge initial impressions. It’s an excellent way to test assumptions from discovery. On key screens we even make elements interactive so they could give more detailed feedback.
Build - Finally we have something live for folks to play with. We create preview links in the production app and have grant writers try it out while sharing their screen. The key to doing this well is setting clear context and iterating on feedback quickly: every week, we come with new prototypes and designs based on our learnings.
If you are building out your own process, remember the most important rule to receiving good feedback: tell people what you want feedback on.
What have we learned?
Our biggest learning thus far: Remove Magic to build trust in generative applications.
What does this mean?
AI-powered products often feel magical, however, when the use case requires accuracy, generated content is more terrifying than useful: not knowing where something comes from makes it impossible to trust. This is an age-old problem in AI, commonly referred to as AI explainability or AI interpretability. In our case, we help draft Letters of Intent, Project Plans, etc. This text is technical and cannot have mistakes. Naturally, writers are worried about accuracy of generated text!
To combat this, we’ve intentionally peeled back the layers of AI Generation to expose what’s under the hood, removing magic almost entirely. In the example below, we show the sources that are inserted into generation along with the prompt itself. When there’s a lack of confidence, we show that as well, requesting more information.

In the photo above, you can see how Streamline identifies relevant sources from uploaded documents and shows this to users in the context of the overall document before we attempt to generate a paragraph.
Below, see how sources can easily be added, excluded, or even modified. Streamline also makes it easy to add context in the shape of bullet points.
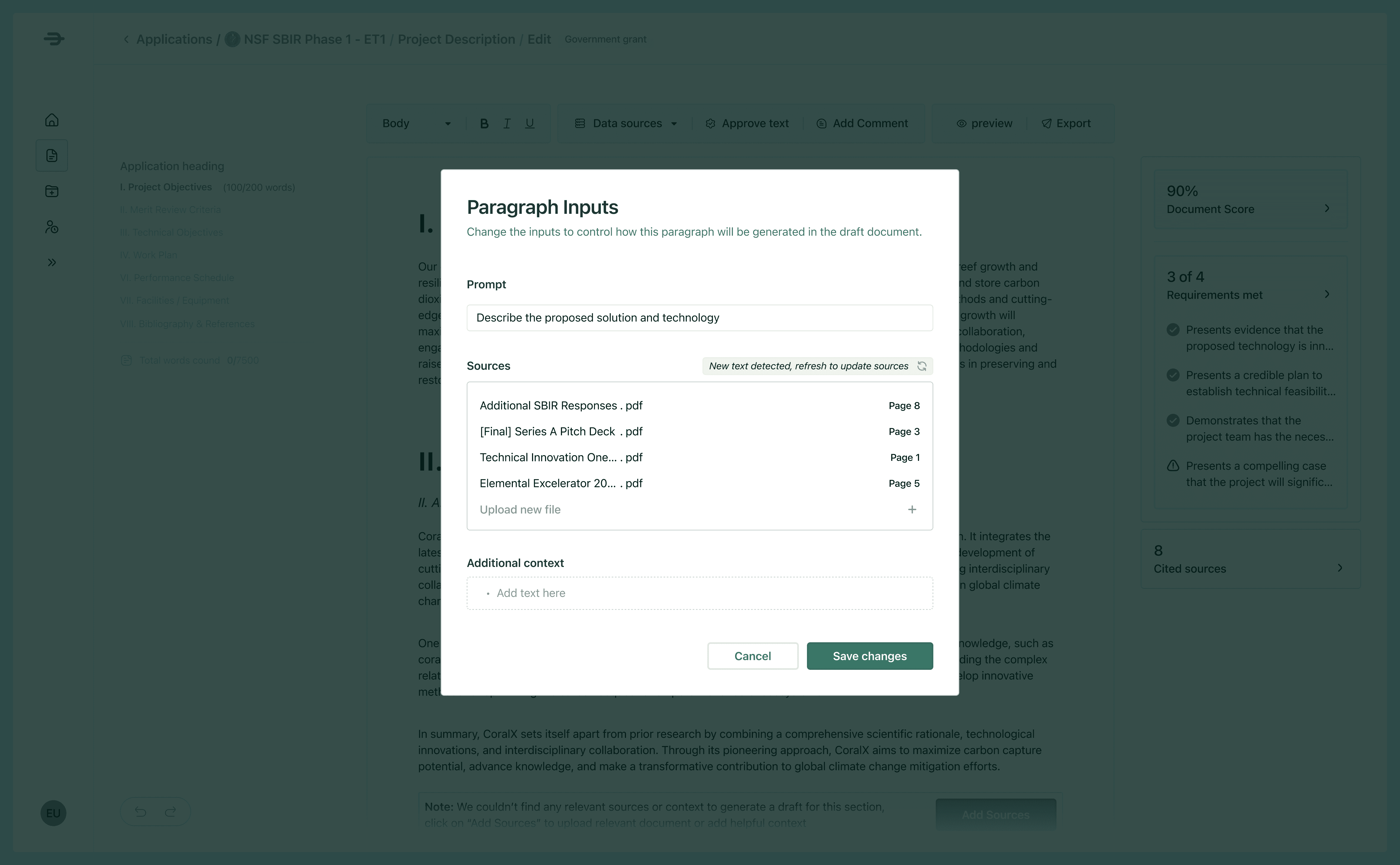
In the next few weeks, we’ll be doing deep dives on our upcoming features and the inspiration behind them.
What’s next?
We are excited to launch the government grants product in beta. Grab a time with our founder to get onboarded — assuming you’re ready to play with something before it’s fully polished.
In the next few weeks, we’ll dive into specific features in our new product for government grants inspired by each of our design partners: